In this data-driven environment, many decisions and communications happen, making it complicated to organize and understand huge amounts of information. Knowledge graphs, therefore, help structure information into an interconnected set of data points, or ‘nodes’ , in which knowledge can be represented that machines can understand and leverage. This article explores the evolution, construction, techniques, applications, challenges, and future trends of knowledge graphs.
The Evolution of Knowledge Graphs
Knowledge graphs are not a new concept. Early representations of knowledge started with the semantic networks and frame-based systems of the 1970s and 1980s, that used nodes (representing entities) and edges (representing relationships) to model knowledge, but could not scale well and were generally too narrow in scope.
A lot of buzz was witnessed around 2012 when Google rolled out its Knowledge Graph, a huge database that it expected would make its search engine better at connecting the appropriate entities. This way, Google would turn back ever more intelligent answers to even more questions of its users by establishing relationships between ideas such as “Leonardo da Vinci,” for instance, being not just a painter but also an inventor. Other tech giants, like Microsoft with Bing’s Satori and Facebook with its Graph API, continued on the same trend and further accelerated the adoption of knowledge graphs as an enabler to support search engines, recommendations, and other social networking services.
How Knowledge Graphs Work
A knowledge graph can be represented as nodes, edges, and properties that describe information about entities such as people, places, or concepts, along with the relationship between the entities. For example, in a knowledge graph of movies, a node would represent the movie Inception, and some edge would represent the relationship between the film Inception and its director, Christopher Nolan, with further properties describing the release year, the genre, or the box office revenue of the film.
The Role of Ontology in Defining Structure
Secondly, there is the issue of ontology. Ontology defines a schema and structure dictating how information is categorized. Categories describe entities, their relationships, and the rules governing them. This explains consistency regarding data representation. All information within the graph is, therefore, said to be associated with some predefined structure. This is critical to ensure that data coming from several sources may be combined and, hence, intelligible.
Data Integration, Mapping, and Linking
It refers to the inclusion of data from different sources into a united knowledge graph. Data mapping is the interpretation of data in one schema or model into another, while ensuring that the translated information matches the ontology of the graph. Data linking refers to how disconnected points of data are connected, ensuring that entities between different data sets get correlated properly, such as linking an actor’s profile in one database with a movie’s information in another.
Major Techniques in Building Knowledge Graphs
The construction of a knowledge graph involves many sophisticated techniques, each providing significant contributions to the correctness, completeness, and utility of the graph.
Entity Recognition and Linking
This technique discovers entities, such as people, places, or organizations, that might exist within unstructured data, like text. Following their detection, these entities may be merged with the nodes already in place within the knowledge graph to remove redundancy.
Data Ingestion
Gathering and processing data from different sources, which can be structured from databases, semi-structured from XML or JSON, or derived from articles or social media posts, which are presented in unstructured forms.
Graph Analytics
This uses the ingested and cleaned data to discover patterns and insights in the form of discovering clusters of related entities or the shortest path between two nodes in the graph.
Relationship Extraction
Extract all of the relationships between entities. For example, parsing text to determine that “Steve Jobs” founded “Apple” and connect these two entities in the graph with a “founded” relationship.
Applications of Knowledge Graphs
Knowledge graphs have manifold applications in every imaginable industry:
Healthcare
The knowledge graph can be used in the health industry to represent complicated relationships between diseases, treatments, symptoms, and drugs. It might be useful in guiding doctors or researchers to treatment pathways or creating patterns regarding patient data.
Finance
Banks use knowledge graphs to understand the relationship between the clients and the transactions, as well as the trends existing in the market. It helps in detecting fraud by analyzing the intricate web of connections between the accounts and the transactions.
Search Engines
Search engines use such structures, like Google’s Knowledge Graph, to return much more informed and accurate results. When a user queries for a term such as “Albert Einstein,” graph entities involved in his biography, work, and contributions, aside from awards, are linked for a rich summary instead of a list of links.
Sobot.io and Knowledge Graphs
Relevant solutions for knowledge graphs can be smartly utilized on platforms like Sobot.io. We create understandings of queries in relation to the context and the relationship between objects, solely relying on the most updated algorithms in machine learning, and deliver the appropriate responses to customers. Integration with knowledge graphs will make these understandings regarding context and the relationship between objects more accurate and personalized in terms of customer interaction.
Challenges in Building and Maintaining Knowledge Graphs
Though there are several advantages of knowledge graphs, there are various challenges associated with the creation and maintenance of knowledge graphs.
Scalability
The practical challenge for such growth continues to be scaling knowledge graphs to support millions or billions of nodes and their relationships. The larger the graph, the more computationally expensive queries are.
Data privacy
With the proliferation of data-centric technologies, issues of data privacy arise. Heterogeneous data sources are inherently linked by knowledge graphs, which can lead to violations of privacy if managed recklessly.
Data Inconsistency
Data within large, integrated systems may contain inconsistencies. Data from different sources might not be consistent. Different types of entities in disparate databases could be different, or even the relations between two entities could contradict other sources. Considering a knowledge graph requires continuous supervision and updating to maintain consistency and accuracy.
Future Trends in Knowledge Graphs
Looking forward, the future of knowledge graphs is promising, as several emerging trends are going to shape their development further:
Automated Generation
With the advancements in AI and ML, knowledge graph generation is increasingly becoming automated. AI can be used to identify entities and relationships to ensure that the knowledge graph stays updated with current information.
Integration of knowledge graphs with AI and ML
The integration of knowledge graphs with AI and ML models is becoming increasingly popular. The incorporation of knowledge graphs in an AI system allows AI systems to understand and reason the world with better structure. Hence, the decision-making and reasoning capabilities of such systems improve, and better predictions arising from the knowledge graph can be obtained for one kind of machine learning model.
Knowledge graphs evolved from early knowledge representation systems to become one of the cornerstones of modern data management and integration. They allow for connecting different pieces of information into a unified structure and can support many different applications, search engines, and industries, from healthcare to finance. However, among other problems, scalability, data privacy, and inconsistency remain. Overall, knowledge graphs will be much more powerful with all these advanced works done on automated generation and integration with AI.
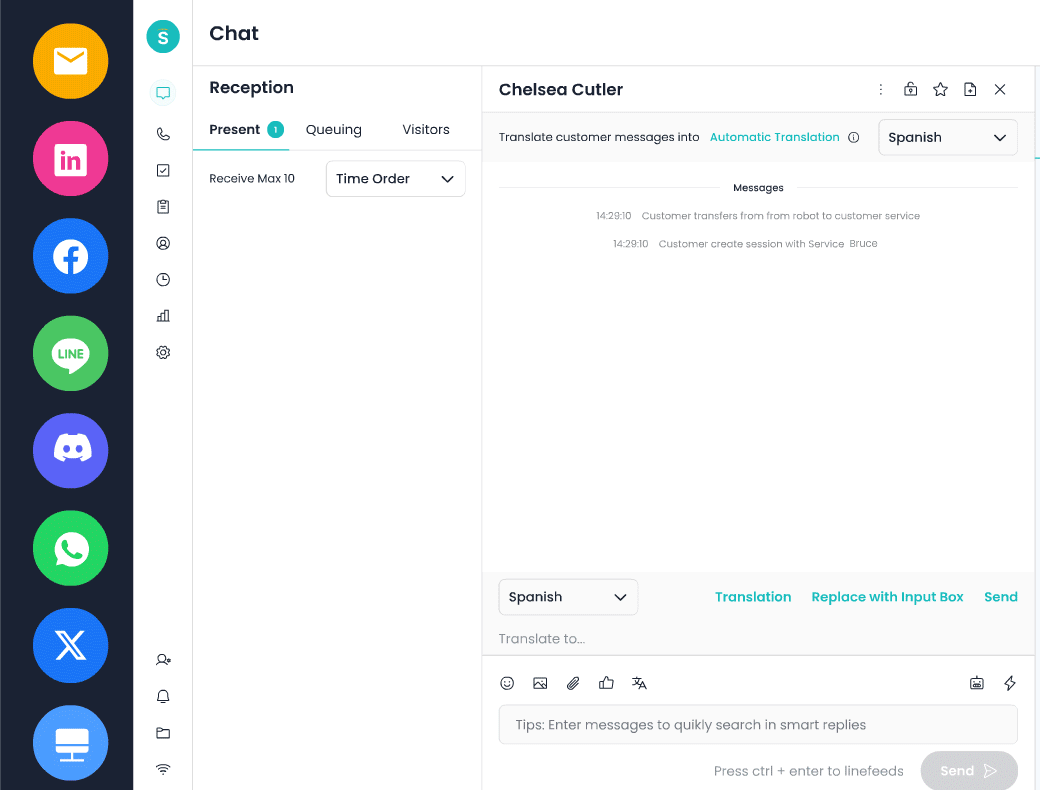