Named Entity Recognition, or simply NER can be described as the process of identifying and classifying key entities within the text. The most common categories of key entities are names of people, organizations, locations, dates, monetary values, and so on. NER means transforming unstructured data into structured forms for easier machine analysis and interpretation. NER has now become an increasingly vital application in search engines, chatbots, and in monitoring of different social media channels as the generation of data exploded on these numerous platforms. Still, it continues to support such applications by automatically extracting relevant information from large corpora of texts to improve data usability and facilitate informed decision-making at organizational levels.
How Does NER Work?
First, the text must undergo preprocessing; this is the first step in performing the Named Entity Recognition process. It is a significant step to take raw text and prepare it for analysis. The preprocessing stage typically involves several steps, including deleting unused characters and extraneous punctuation and information to clean up the text, which makes the context around the entities more transparent. Then comes tokenization, where the text is broken into small units like words, phrases, etc., that are called tokens. Then, it’s analyzed to see if a particular token represents an entity. For example: “Microsoft announced a partnership with OpenAI,” “Microsoft” and “OpenAI” would be labelled as organizations.
After performing the tokenization step, the next crucial phase is model training. The phase trains a basic machine learning entity recognition model to identify and categorize entities from the dataset. Labelled datasets are text datasets given here where entities are identified and categorized. Some popular NER datasets are CoNLL-2003 and OntoNotes, which offer comprehensive examples within many categories. This model uses different algorithms to learn patterns and relationships between words. These allow the model to generalize and predict unseen data.
After training, sequence labelling algorithms extract the entities in the sentence. Traditionally, the models of CRFs and HMMs were widely used. However, it brought even more complex models, such as combining Bidirectional Long Short-Term Memory (BiLSTM) networks with CRF layers and Transformer-based models like BERT.
Types of Entities Recognized by NER Models
NER models are designed to recognize all forms of entities. The most general categories include people or persons, which describe proper names referring to human beings, organizations, and other legal entities. Locations refer to geographically meaningful entities, including cities (“New York”), countries (“India”), and places (“Eiffel Tower”). Important date and time expressions enable a better sense of the context of the events. Further NER models identify monetary values and numerical quantities that prove helpful in financial applications. The other traditional categories are recognized; however, miscellaneous entities, which may include products, events, and artistic works, are recognized by some NER models depending on the application and the specific focus of the domain.
Techniques and Models for NER
NER systems use quite disparate techniques in most general approaches and can be broadly classified under rule-based NER, machine learning-based NER, and deep learning-based NER. In rule-based systems, predefined sets of rules and dictionaries are used to identify an entity. This approach basically relies on expert knowledge to create rules based on patterns and syntax. Although a rule-based system may be very successful for a particular application, it lacks flexibility compared to the complexities and nuances of natural language.
Machine learning-based NER uses algorithms to learn patterns from labeled datasets, which enables the system to generalize much better than in rule-based approaches. SVMs and CRFs have been widely used techniques for NER tasks. They learned from the data and adjusted their parameters to better predict accuracy.
Deep learning has now been applied in the latest NER systems. Models like BERT, ELMo, and GPT have deep neural networks that learn from significant texts. Such models capture the complexity in language by considering the context in which a word appears, so they are broadly suitable for the identification of entities in complex sentences. NER systems based on deep learning often result in better performance, but they do require high computational resources and large labeled datasets to train efficiently.
Challenges in NER
Though very useful, NER has several disadvantages. The most prominent one is the vagueness associated with most of the words. Usually, a single word will have multiple meanings depending on the context. “Apple” may refer to the fruit or the technology giant.
Another challenge is the language of different domains. Models trained on general datasets may perform poorly in identifying entities in certain field-related domains, such as medicine or law, where every domain has peculiar jargon and terminology. To overcome this challenge, we must develop, train, and fine-tune the models using relevant data within their particular areas of interest.
Others include handling OOV entities. NER models do not handle newly introduced names, terms, and acronyms, failing to recognize them because their training was at a different time.
Applications of NER
NER is used in many places and has applications in various industries. As far as information retrieval systems are concerned, NER bolsters the overall effectiveness of search engines as it identifies explicitly key entities in the user’s query and, therefore, provides highly relevant responses to such queries. For example, if a user submits a query that asks to “seek restaurants in New York,” NER would identify that “New York” is indeed a location.
NER is very important in sentiment analysis because a company can extract products, brands, or public figures from social media and reviews, allowing it to measure its standings and accept necessary corrections. For instance, a company can track the social media services for its products so it may better learn what customers think of them and what they prefer.
In customer services, NER has helped chatbots and virtual assistants understand questions better than earlier. Such systems identify the names of products, locations, etc., so correct answers are returned, and procedures for customer interaction are simplified.
In addition, it assists in crucial contributions in healthcare by extracting essential information concerning the patient from medical records and clinical notes. This capability promotes the diagnosis, treatment, and reporting process through the facilitation of critical organizing that can support healthcare professionals in making adequate decisions.
The Future of NER
The bright future of NER development is tied to such improvements in AI and deep machine learning: new innovations in zero-shot and few-shot learning techniques will break the dependence on big labeled datasets, making models function better across various domains. Such approaches allow for making predictions about entities that a NER system has not experienced before, thus making its use significantly more adaptive.
In addition, knowledge graphs also allow for the introduction of NER because knowledge graphs represent relationships between entities, thus providing a better view of holistic information extracted by NER. This will provide a more contextual understanding of entities and their interrelations.
As the requirement to process text data continuously in real time continues to skyrocket, it will play a salient role in any smart device and IoT system that generates unstructured data on a huge scale. To put it differently, these advances demand NER models capable of withstanding sustained data processing and analysis at hitherto unheard-of speeds.
Named Entity Recognition is a potent tool for extracting meaningful information from unstructured data. The tool stretches across several industrial sectors from its applications, increasing efficiency and improving user experience. With such challenges as ambiguity, domain specificity, and multilingualism, ongoing research and technological advancements are well-suited to enhance NER capabilities further. As NER continues to evolve, it will have a critical role in shaping the future of intelligent information systems, therefore contributing to the ever-growing field of Natural Language Processing and the applications its various areas have made in diverse sectors.
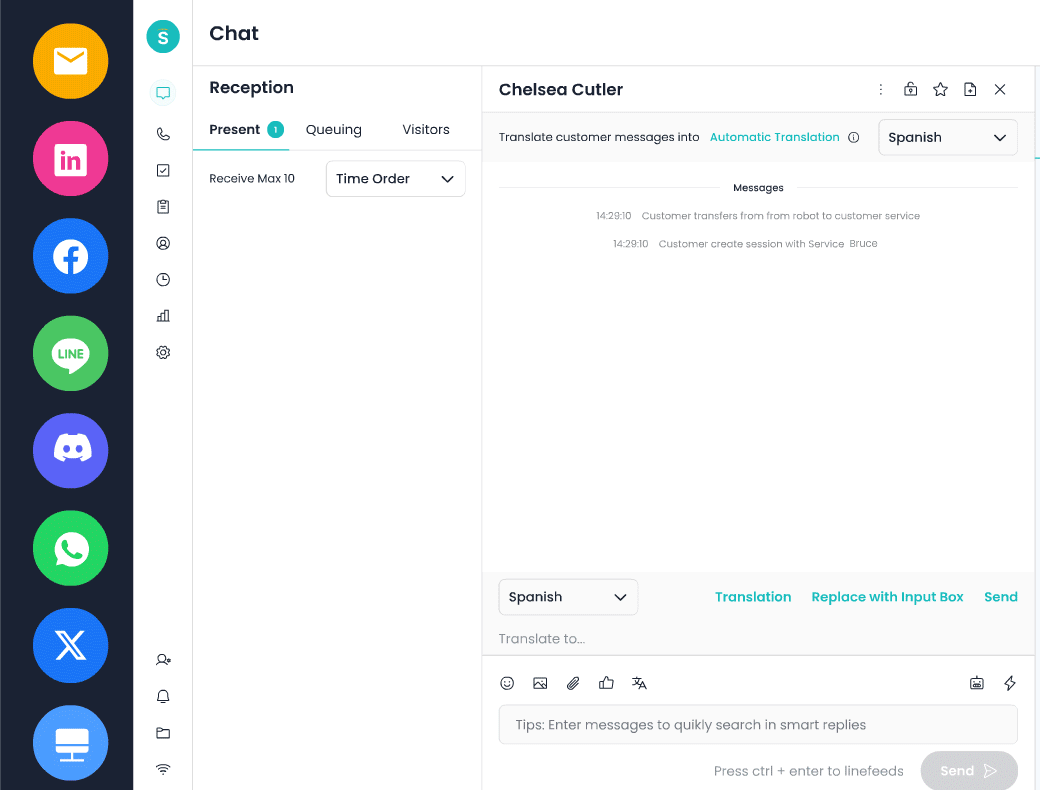